From Chaos to Clarity: Proposal Evaluations in Web3 Grant Programs
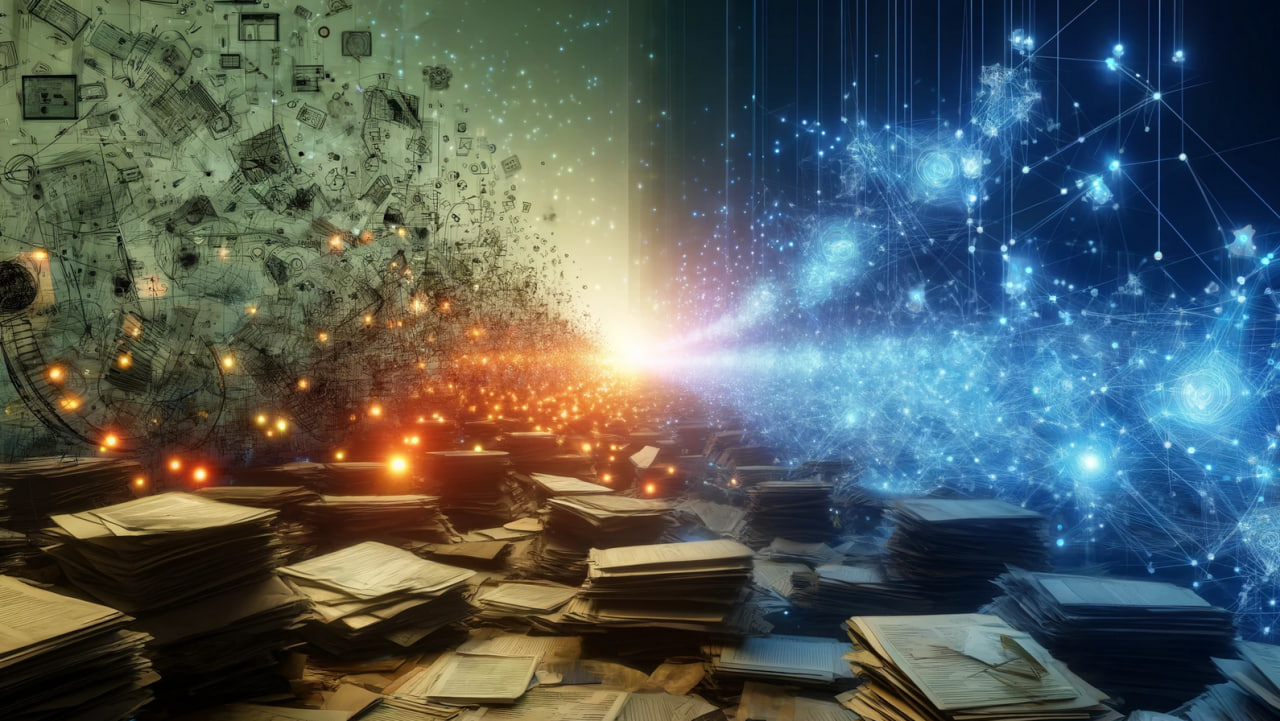
TL;DR
This article addresses the challenges of allocating funds within Web3 grant programs and highlights the need for a more reliable evaluation process for project proposals and outcomes. With the growth and innovation of the Web3 ecosystem at stake, it is critical that grants reach projects with real impact potential. The paper outlines the complexity of the current funding processes of different programs, which range from community-led to expert-led evaluations and highlights the shortcomings of existing evaluation methods.
Experience has shown that expert-led programs often depend on a small, overburdened group to provide the necessary depth and breadth of evaluation, while in community-led programs, the lack of expertise to evaluate these projects increases as the complexity of the projects increases. This often results in generally unreliable evaluations, which leads to well-known applicants being favored and potentially groundbreaking projects being overlooked
A reputation-based review system (RRS) is proposed as a suggestion for further research. This system aims to identify the reviewers who have provided reliable evaluations in the past. This means that in addition to the project outcome and impact, the original evaluation of the proposal is also re-evaluated. The reviewers with reliable past evaluations are given more weight in subsequent rounds, which is expected to result in a better selection of projects.
The importance of refining evaluation processes goes beyond the realm of Web3 grants and touches on broader issues of review and information reliability in various sectors, including e-commerce, academic research and news media. The paper suggests that the implementation of reputation systems could play a central role in ensuring the integrity and reliability of information in the digital age, and calls for further research and experimentation in this direction.
Introduction
What is it about?
In the rapidly evolving Web3 landscape, the allocation of funds within grant programs has become a critical point of contention, sparking debate and raising concerns about the effectiveness of these initiatives. With billions of dollars at stake, the question of how these funds are allocated and, more importantly, whether they reach the projects that can truly catalyze growth and innovation within the ecosystem has never been more relevant.
In light of the recent criticism of several leading funding programs, the need for a more reliable, transparent and accountable evaluation process for project proposals and outcomes is apparent. I would therefore like to use this paper to examine this problem of unreliable evaluations and reviews in more detail and show a possible way to make these evaluation processes more reliable.
Why is this topic important?
The importance of this topic lies in the central role that the proposal evaluation process plays in grant programs, as it lays the foundation for the selection of the most promising proposals. Reliable evaluations are crucial, in grant programs, but also in many digital areas like research and journalism Inaccurate evaluations can lead to resources being misallocated and misinformation being spread. This exploration of grant programs is only the beginning, as various other areas are also confronted with this challenge, which shows the far-reaching effects of unreliable evaluation processes and information.
Context: What are Web3 Grant Programs?
Web3 grant programs are typically sponsored by blockchain organizations to fund the growth of their ecosystem. These programs have different focuses, structures and degrees of decentralization. The funding process is usually as follows:
- A funding round is announced with a specific funding goal.
- The application process is open and freely accessible to any team that wants to submit a proposal.
- Proposals are evaluated by the entire community, an elected committee or a selected team of experts.
- The decision on which proposals receive funding is made either by the entire community (token holders), an elected committee or a selected team of experts.
These grants are critical to the advancement of the decentralized web, as they support a variety of projects that drive innovation and contribute to a user-centric Internet. The degree of decentralization in the decision-making in these programs varies, ranging from expert-led to community-driven processes. Grant amounts are typically between $1,000 and $500,000 and range from specific community projects to broader open-source projects.
Understanding the problem
A recent report by Mashal Waqar and Eugene Leventhal on Web3 grant programs and the feedback I received from program managers reveal critical flaws in the evaluation processes within Web3 grant programs, particularly in evaluating the feasibility and potential impact of project proposals, and later, their actual impact. The emergence of grant farming, for example, shows how difficult it is to assess the true capabilities of proposers and highlights the consequences of unreliable reviews: misallocated funds and stifled innovation.
Problem Statement as I believe it today
I am a decision maker in a Web3 grant program, and I am trying to select the best proposals to receive funds, but it’s hard to evaluate the feasibility and potential impact of all project proposals, because there are too many projects to review and available evaluations of the projects are often not reliable, which makes me feel overwhelmed and frustrated.
Expert Review Limitations
In many Web3 grant programs, instead of a fully decentralized proposal evaluation process (i.e. anyone from the community can evaluate projects), a committee or team of experts is set up to evaluate the proposals, and sometimes even decide which proposals are awarded or at least give some kind of recommendation to the community. Using experts for the evaluation process can lead to better results in the short term, but it does not scale as the number of projects increases and the number of experts is limited. Many programs have fewer than 10 reviewers, for example. This means that a large number of projects has overwhelmed reviewers of many programs, as can be seen from the NEAR’s review team or the recent feedback from the reviewers (called badgeholders) of Optimism’s Retro Fund 3.
However, a limited number of reviewers not only limits the number of good reviews, but also the expertise, as the projects cover more and more areas and sometimes require very specific expertise. For example, code review can be very time-consuming and specialized. In one case, I spoke to a manager of a grant program who told me that there were teams that submitted only code created by ChatGPT, which wasn’t detected in the flood of proposals.
As programs get bigger and more diverse, it becomes increasingly important to reliably evaluate the work of the committees and experts as well. And this ends up going round in circles, because the experts have to be evaluated by other experts. In other words, selected committees and experts can be helpful, but often do not scale.
Impact Assessment Challenges
In Web3 funding, the mirror image of evaluation of proposals is evaluation of the impact achieved by the projects. The information provided by the projects is often insufficient to make an accurate assessment of their impact, which often leads to subjective rather than data-driven impact evaluations. At the same time, it is also recognized that measuring hard KPIs like on-chain data is often ineffective, because these numbers often do not accurately reflect the engagement or impact generated by these projects. This means that, on the one hand, we need subjective assessments, but on the other hand, because these assessments are not systematically and verifiably captured, they lead to biased and inaccurate evaluations.
In addition, it is also important to track the correct use of funds, as there are always cases of projects being suspected to not use their funds properly, which is very costly to investigate in detail. Furthermore, in some ecosystems it has been difficult to accurately determine the actual impact not only at the proposal level but also at the program level, resulting in some programs being discontinued or paused, such as Polygon.
The pain points of the proposal reviewing process in community-driven programs
Open review systems (i.e. anyone from the community has the opportunity to evaluate projects) have the advantage that there is a large number of reviewers, but this often goes hand in hand with a flood of information that is difficult to verify. The quality of evaluations can vary considerably due to the anonymity and varying expertise of the reviewers, which undermines the reliability of the proposal evaluations. The following difficulties can be observed:
- Decentralization vs. quality control: Web3 and decentralized ecosystems promote openness and participation from a wide array of contributors. However, this decentralization complicates efforts to enforce consistent quality control and verification mechanisms for reviews without central authority.
- Varying expertise: The evaluations come from a variety of people with different levels of knowledge and experience, which affects the quality and therefore the relevance of the evaluations.
- Complexity of proposals: The technical and innovative nature of projects in Web3 Grant programs means evaluating them requires specialized knowledge. This complexity makes it difficult for a broad reviewer base to provide informed and reliable assessments consistently.
- Bias and subjectivity: Personal bias can influence reviewers and result in ratings that may not accurately reflect the value of a project.
- Anonymity and accountability: Anonymity limits the accountability of reviewers, which jeopardizes the quality and integrity of reviews.
- Incentive misalignment: Current incentive structures often do not align with the goal of producing high-quality, reliable reviews. Reviewers may be motivated by rewards that do not necessarily correlate with the thoroughness or accuracy of their reviews, leading to superficial or biased evaluations.
This results in the following:
If the reliability of evaluations is diluted, it is difficult for voters to recognize which projects really deserve support. And instead of being supported in their decision-making process, voters now have to evaluate the projects for themselves:
- Voter information overload: The volume and complexity of proposals make it almost impossible for voters to thoroughly evaluate each proposal, which can lead to deserving projects being overlooked and an over-reliance on superficial indicators.
- The problem of proposer visibility: In a scenario where evaluations are unreliable and voters overwhelmed, funding decisions can degenerate into popularity contests. Moreover, the visibility of proposers in an ecosystem becomes a critical factor, so decisions are made in favor of those who talk rather than build, which is a disadvantage for lesser-known innovators.
Zooming out
In the next section, I want to take a quick step back and see that the challenge of reliable evaluations is not just a problem of grant programs, but a general problem in the digital world. If we look at other industries and use cases, we can see some parallels:
- Evaluating Products in E-Commerce: Here we see a large number of fake reviews and the general trend that reviews of products and services are becoming less and less important because they are increasingly seen as unreliable.
- Evaluating Research in Academia: The peer review process is essential to ensure the reliability of published work. However, biased peer reviews and often non-transparent review processes jeopardize the foundation of scientific work, as studies cannot be independently verified, leading to the dissemination of unreliable or erroneous research results, especially in medical research.
- Evaluating Borrowers in DeFi (Decentralized Finance): Unreliable assessments of borrowers and lending protocols can cause lenders to lose significant investments or prevent new borrowers from entering the sector.
- Evaluating Reports in News Media: The reliability of news and reports is under constant threat from misinformation, biased reporting, and sensationalism, fueled by the race to attract as many viewers as possible which undermines public trust and the fundamental role of journalism in democracy.
- Evaluating Governance Proposals in DAOs: In the unique ecosystem of DAOs, where governance is decentralized and decisions are made collectively based on voting, the accuracy and credibility of information are critical. Misleading data or flawed verification processes can skew voting results, distort the evaluation of proposals and inaccurately represent members’ contributions.
- Evaluating Contributors and their Work in Collaborative Projects: Especially in remote or decentralized environments, the reliability and authenticity of contributors and their contributions are critical. Misrepresentation of skills or false evaluations of contributions reduces project quality and affects morale and trust within teams.
The list is probably longer and growing. From security audits to new developments in the blockchain industry like consumer social and NFTfi. If we take a closer look at these use cases, we can identify some of the main causes for the unreliability evaluations of information:
- Information Overload: The overwhelming amount of online data, combined with the complexity of certain areas, makes it all but impossible for an individual to accurately evaluate or categorize a single piece of information, leading to an inability to distinguish potentially unreliable sources from reliable ones.
- Lack of Verification Mechanisms: Without robust systems to verify the authenticity and accuracy of information or the reliability of sources, digital platforms are vulnerable to the spread of misinformation. Biased or deliberately misleading reviews, whether in academic research, decentralized innovation funds or e-commerce, undermine their reliability and can skew perceptions and lead to the promotion of undeserving work or products.
- Anonymity and Lack of Accountability: The anonymity afforded by digital platforms, while promoting freedom and participation, often comes at the cost of accountability. This allows individuals to spread false information or engage in deceptive practices without direct repercussions.
- Counter-productive Economic Incentives: The drive for clicks, views, and sales can incentivize the creation and dissemination of sensational or outright false information. This is particularly evident in the news industry and e-commerce, where sensationalism and fake reviews can significantly impact consumer behavior.
Seeing the market
It is important to estimate the market size of ensuring the reliability of reviews within Web3 grant programs. While this is a rather theoretical undertaking, even rough guesses show us its market potential. For the calculation, we consider digital public goods as the Total Addressable Market (TAM) and Web3 funding programs as the Serviceable Available Market (SAM). The limitation is that some Web3 grants are also awarded for venture activities.
The approach used here is to first estimate the total volume of funding for digital public goods and for Web3 grants and assume that a small percentage (depending on the use case) between 0.1% and 1% of the total market is dedicated to the evaluation of projects in order to increase the funding effectiveness.
Total Addressable Market (TAM) — Digital Public Goods
Digital Public Goods (DPGs), encompassing open-source software, open data, open AI models, open standards, and open content, represent a rapidly growing sector within the global digital ecosystem. The Digital Public Goods Alliance (DPGA) highlights their increasing importance in fostering innovation, transparency, and accessibility across various fields. Despite being a relatively new market, DPGs are projected to reach a market value of approximately USD 100 billion annually by 2030, according to forecasts by Gartner and EY. Assuming that an amount of at least 0.1% of the total volume is used for the evaluation of proposals in order to better allocate the funds, this would already mean a market of over 100 million dollars.
The choice of DPGs as a TAM is made for several reasons: Both DPGs and Web3 share a common ethos of open access, collaboration, and democratization of digital resources, making them naturally aligned. This approach also demonstrates the interlinked growth opportunities between Web3 and other open digital initiatives.
Serviceable Available Market (SAM) — Web3 Grant Programs
Assuming Web3 grant programs could capture or influence a segment of the broader public good funding towards digital public goods, the SAM could be conservatively estimated in the range of potentially several billion dollars. This estimation considers current funding trends, the increasing adoption of blockchain technology, and the expanding definition of digital public goods.
Specific annual funding amounts are difficult to determine due to the ecosystem still being developed and constantly changing. Currently there are over 170 active grant programs. My rough estimate from this is that between $ 0.5–1 billion will be distributed in grants in 2024.
Assuming that programs could spend 1% of their funds on ensuring the reliability of project proposals and outcomes through, the SAM for related verification services in this area can be estimated to be $5–10 million annually.
It’s important to recognize the theoretical nature of this market sizing and the reliance on available data and informed assumptions. The actual figures for both TAM and SAM may fluctuate as more precise information becomes available and as the Web3 ecosystem continues to evolve.
Map of the space
The landscape of Web 3 grant programs is very scattered and is developing and changing at a rapid pace, making the creation of an up-to-date map a challenge in itself. And this applies even more to the approaches, ideas and projects surrounding governance and the processes for selection and evaluation projects.
However, a few fundamental observations can be made:
- It is still a very experimental space. New programs or funding rounds are announced almost weekly, promising an improved process and better outcomes.
- In response to the variety and diversity of projects, many ecosystems have a whole range of programs.
- The search for the right financing and evaluation models is in full swing and ranges from the complete delegation of review and selection tasks to the community to total reliance on internal staff or hired experts.
- Many ecosystems are struggling with the fact that a large proportion of previously funded projects are no longer active as seen in Polkadot, for example.
- The incentives offered to the community for active participation in the funding process are constantly changed, removed and reintroduced in both the review and selection process.
In short, incentives that are too low lead to a decline in community engagement because efforts and expertise are not adequately rewarded. If incentives are too high, the risk of systems being exploited or cheated increases.
In addition to the above-mentioned report by Mashal Waqar and Eugene Leventhal, the following is intended to present some current developments in major grant programs that illustrate the challenges of reliable proposal evaluations:
Arbitrum: Arbitrum DAO plans to launch a long-term incentive pilot program and payout up to $90 million in ARB to improve Layer 2 network development and user base. This program aims to address past challenges by introducing a five-member council and three advisors to review proposals and provide feedback, and is intended to reduce the burden on delegates and encourage more innovative incentive mechanisms. The last STIP round (Short Term Incentive Program) experienced some problems in its implementation, especially during the voting phase. The tight time frame for evaluating and voting on numerous proposals highlighted the importance of thorough evaluation within the governance framework.
Cardano: As an active participant in Project Catalyst (Cardano’s Grant Program), I have been involved as a proposer, reviewer, and voter in all rounds from the very beginning. From my personal experience and a multitude of conversations and interactions with other participants, I have been able to observe the challenges and the evolution of a highly community-driven approach very closely. Even though I have a lot of respect for the fact that a community approach has been chosen and do not overlook the progress made, especially in the last round, it is still the case that the proposal reviews are not really used by many voters as a reliable tool for identifying the best projects, as the quality of the individual reviewers can only be determined with a great deal of effort. Even as a successful proposer in the last round (Fund 11), I find the quality of many reviews questionable, because they are either very general and unspecific or have obvious opinions or argumentative gaps.
Optimism: Optimism’s Retro funding grant program has recently come under criticism from the community for seemingly favoring large or more established projects over smaller projects in some cases. There, the evaluation and selection process is carried out by so-called badgeholders (mostly people elected by the community). However, many of them were unable to cope with the number of proposals, which resulted in the aforementioned bias of favoring well-known projects. Recent developments have increasingly focused on verifiable impacts and the shift to more closely grouped and topic-specific rounds, but still suffer from the problems of evaluating the impact itself, which I discuss below.
Polygon: Polygon’s previous grant program has faced its own set of challenges, particularly in assessing the genuine impact of funded projects and ensuring the effective utilization of disbursed funds. Similar to the concerns raised in Optimism’s community, there have been instances within the Polygon ecosystem where the alignment between the goals of funded projects and their actual contributions to the ecosystem was questioned. As a result, the program was stopped and the effectiveness of the newly introduced approach has yet to be determined.
Why it hasn’t been solved yet
The reliability of the evaluation of proposals is repeatedly compromised by the fact that there are no generally recognized standards — both for the evaluation of proposals and for the assessment of their impact. As a result, different evaluators may prioritize different aspects of a proposal, leading to evaluations that lack consistency and uniformity.
To make matters worse, it is difficult to reach consensus within decentralized communities on the adoption of standardized evaluation criteria and review systems. Differing views on how assessments should be conducted, who should oversee them and what the framework for rewards and sanctions should look like add to this complexity. Moreover, even initiatives that have worked hard to quantify impact evaluations in recent years still face challenges in measuring them meaningfully.
The question of how to objectively assess impact has not yet been answered. The problem with subjective evaluations of proposals in Web3 funding programs, or indeed in many evaluation processes, is that their inherent variability makes it difficult to create and ensure a fair and accurate overall picture through models and algorithms. This variability undermines the ability to produce a comprehensive and accurate representation of the value and potential of proposals and makes it increasingly difficult to manage and refine evaluation processes in a way that is consistent and accurate.
Another question that arises here is whether the aggregation of subjective opinions can come close to an objective truth. This phenomenon, often referred to as the “wisdom of crowds”, means that under certain conditions the average of many people’s guesses or opinions can be surprisingly accurate. While aggregating subjective assessments can lead to a more reliable and standardized form of evaluation, it does not make the assessments truly objective in itself. Instead, it could provide a consensus-based approach that can serve as a practical substitute for objectivity under the right conditions.
In academia and scientific research, peer review involves the evaluation of the quality, relevance and accuracy of a scientific paper by multiple experts. Although each evaluation is subjective, the aggregation of these assessments can help to ensure the credibility and validity of the work.
What success will look like
To better understand the extent of the problem, the following is a brief outline of how a reliable evaluation process would affect participants in grant programs, such as proposer, reviewer and voter:
- Better use of funds: Reliable project evaluations can ensure that proposers and projects are identified whose ideas and contributions are most likely to achieve the set fund goals.
- Easier and better decision-making: With reliable evaluations, voters can make more informed decisions and select proposals that promise the greatest value and potential for the ecosystem.
- Better Feedback: For proposers, reliable evaluations from reviewers could lead them to seek feedback from credible sources, improving the quality and approval rate of their proposals, and eventually the success rate of their projects.
- Higher participation rate: Reliable assessments could lower the barriers to entry for new participants by creating a level playing field for new and unknown teams and projects.
The following metrics could be used to measure this success:
- Engagement: an increase in active participation from proposers, reviewers and members could show the success of the rating system.
- Efficient funding: The percentage of projects that successfully reach their milestones or deliver the expected results after funding, indicating better targeting of funds to high potential projects.
- Funding distribution: Analyzing the distribution of new and established projects among participants with the goal of achieving a balanced distribution that reflects a healthy ecosystem with opportunities for growth and recognition.
Recommendations
The following is a list of requirements that could be included in future iterations for evaluation processes in grant programs:
The goal must be to create a system that promotes high quality reviewers and reduces the possibility of gaming the system.
To achieve this, the following aspects should be considered and guaranteed in the evaluation process:
- Transparency: It must be possible to trace who has made which assessments. This can also be done anonymously via DIDs, but it must be possible to draw conclusions about individual activities.
- Incentives: We need an incentive system that fairly rewards excellent and time-consuming work. These can be monetary but also non-monetary (visibility, voting weight, etc.) incentives.
- Openness vs. domain expertise: Decentralized communities thrive through diversity and accessibility. The system should take advantage of this and build a broad and diverse base of experts. At the same time, it must be ensured that reviewers have the knowledge and experience to evaluate a project and its team.
- Reduce bias: There are several ways to reduce reviewer bias, such as randomly assigning which projects are to be reviewed by an individual.
To tackle unreliable reviews in grant programs I would like to introduce a Reputation Based Review System (RRS). The following is a high-level overview and requires further experimentation and investigation.
What is reputation?
Reputation refers to the perceived value or credibility of an entity (individuals, organizations, projects) based on their past actions, contributions, and behaviors within the community. It acts as a trust signal, providing a distilled summary of an entity’s history and the quality of its engagements. In decentralized environments, where traditional centralized authorities or verification mechanisms may be absent or reduced, reputation serves as a critical factor in guiding decisions and improving trust among participants.
Why can reputation be helpful in this context?
In our case, reputation means that we want to evaluate the past performance of the evaluators to identify those who have a good track record, i.e. whose evaluations have contributed to the selection of projects with high impact potential, and these projects have actually achieved this impact. And when we have this data, we can give more weight to those who have given reliable ratings in the past. In other words, a higher reputation will have a higher weighting in the evaluation process. The assumption is that those who have predicted an outcome or expected impact well in the past are more likely to do so in the future.
The question now arises as to who evaluates the reviewers and how this evaluation should be carried out?
The challenge is that this evaluation process also carries the risk of being subjective or biased, as clear benchmarks are not always available. For example, let’s compare a stock analyst who predicts stock prices. This prediction can easily be seen from a single figure, which allows conclusions to be drawn about previous analysis and therefore a clear reputation score can be made. Here, too, it is assumed that someone who has provided good evaluations in the past is also a good evaluator of the current situation. The more data and contributions are collected in a system, the more added value such a system can offer. For example, a stock analyst who has correctly predicted 2 out of 3 prices in the past could have a 2/3 probability of being right in a new prediction. The added value in this case will eventually be that different predictions from different analysts can be compared according to their reputation values.
To mitigate the subjective aspects of evaluating the impact of a project and the judgments of the reviewers, continuous research and the collection of structured data is essential. In other words, a systematic and organized approach to collecting data — with details of who assessed which project and how — is essential to draw conclusions about how reliable an individual assessment was.
Exploratory methods, such as gathering feedback from the community about the perceived impact of a proposal or community satisfaction with project outcomes, could prove invaluable. The aim is to create a comprehensive data set that facilitates the identification of accurate predictions and consequently the recognition of a pool of reliable reviewers. This structured approach aims to refine the evaluation process and increase its reliability and effectiveness.
The same challenge exists in the assessment of domain expertise. Its validation is also an independent assessment process. It is possible for this to be validated by an elected or selected committee. Here too, it is important that this process is also transparent and data-driven so that conclusions can be drawn afterwards about who contributed to good or bad results in this assessment process.
The issue of validation of domain expertise presents a similar hurdle and requires its own evaluation process. A viable approach might involve oversight by a committee, either appointed by election or selection, tasked with verifying the expertise of reviewers. It is critical that this review process is also transparent and also anchored in the data analysis so that subsequent reviews of the committee’s effectiveness in distinguishing between individuals who have positively or negatively influenced the review process are possible.
How to start building and testing a reputation system?
What are the requirements to build a reputation system from scratch and why are Web3 Grant Programs a perfect beachhead market to explore a reputation system in the first place before using it for other use cases and industries?
- Active Communities: To be able to build a reputation system and to make sense at all, it requires an active community whose activities make it possible to build a reputation system in the first place, and in return they can benefit from such a system. And this is already the case in most Web3 ecosystems.
- Clear Metrics: To start a reputation system, you have to define clear metrics about what leads to a high or low reputation. The clearer these metrics are to measure, the better the system works. It is therefore helpful to choose a use case that has easily measurable metrics, especially when starting out. For example, the stock analyst mentioned above can be evaluated more easily than an investigative journalist because reporting may not create a prediction or be tested within a known time... In Web3 programs, we can choose to start with these two metrics: (1) Proposal is on schedule and (2) Proposal is successfully delivered. Once certain hard (measurable) factors are in the system, it becomes easier to integrate soft (subjective) factors such as impact assessments.
- Track Records: When introducing a reputation system, it is helpful if data from past activities is already available, as this allows the quality of the system to be tested against real data and to check whether better decisions would have been made in the past. Many funding programs have been running for several years and therefore have a lot of (often still unstructured) data that can be utilized.
Summary of the presented approach
Structured data collection could allow better conclusions to be drawn about the reliability of past evaluations, which in turn influences the weighting in the current evaluation process and thus, in the best case, predicts better results or provides better recommendations for funding decisions.
For such a system to work, however, it needs either time (because a track record has to be created first) or sufficient data from the past. However, this also means that in theory, it would take more time to manipulate the system, as a positive track record would first have to be created in order to benefit from a higher reputation.
Furthermore, as with every system, a reputation system would have vulnerabilities and opportunities to be exploited, sooner or later. The key point is that it must be cheaper to defend the reliability of the system than to attack it. But this aspect also needs to be further explored and investigated.
How would it change the world?
This paper goes beyond simply analyzing the evaluation processes of grant programs and highlights a key challenge of our time: the erosion of trust in the information that floods our digital landscapes. At a time when artificial intelligence is simplifying and almost infinitely scaling up the creation of content, it has never been more difficult to recognize the credibility of information and its sources. This crisis of reliability threatens the very nature of our digital landscape and makes the search for trustworthy information and reliable reviews more important than ever.
The idea of reputation systems within this discussion is not only a call for innovation in the evaluation process of grant programs, but an approach for a broader proposal in the way we build, maintain, and validate trust in the totality of our digital interactions.
While the outlined approach requires further investigation, the potential impact of a solution in this area can be profound for society. The introduction of reputation mechanisms could open up a new era of digital trust, where information is not only consumed but also critically scrutinized and creators can be rewarded for the authenticity and reliability of their contributions. In this future, the collective search for truth becomes a shared responsibility that can enhance our digital discourse and create a better-informed, more critical and trustworthy digital world.
About the author
Dominik Tilman is the founder of TrustLevel, a project officially launched in 2023 with the mission to improve the reliability of information in the age of AI. This paper is part of the Arbitrum Co.Lab fellowship, in which Dominik further explored the topic of reliability of evaluations in Web3 grant programs.
For more information and updates on TrustLevel and the research on this topic, visit trustlevel.io and follow @dominiktilman on Twitter/X. For direct contact, write to us at mail@dominiktilman(dot)com.
Links and References, here
See Dominik's presentation at our Co.Lab Fellowship Demo Day on April 4th here